Adaptive Kalman Filtering for Postprocessing Ensemble Numerical Weather Predictions
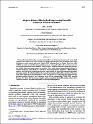
View/ Open
Authors
Pelosi, Anna
Medina, Hanoi
Van den Bergh, Joris
Vannitsem, Stéphane
Battista Chirico, Giovanni
Discipline
Natural sciences
Subject
Automatic weather stations; Ensembles; Forecast verification/skill; Model errors; Model evaluation/performance; Model output statistics
Audience
Scientific
Date
2017-12-04Publisher
AMS
Metadata
Show full item recordDescription
Forecasts from numerical weather prediction models suffer from systematic and nonsystematic errors, which originate from various sources such as subgrid-scale variability affecting large scales. Statistical postprocessing techniques can partly remove such errors. Adaptive MOS techniques based on Kalman filters (here called AMOS), are used to sequentially postprocess the forecasts, by continuously updating the correction parameters as new ground observations become available. These techniques, originally proposed for deterministic forecasts, are valuable when long training datasets do not exist. Here, a new adaptive postprocessing technique for ensemble predictions (called AEMOS) is introduced. The proposed method implements a Kalman filtering approach that fully exploits the information content of the ensemble for updating the parameters of the postprocessing equation. A verification study for the region of Campania in southern Italy is performed. Two years (2014–15) of daily meteorological observations of 10-m wind speed and 2-m temperature from 18 ground-based automatic weather stations are used, comparing them with the corresponding COSMO-LEPS ensemble forecasts. It is shown that the proposed adaptive method outperforms the AMOS method, while it shows comparable results to the member-by-member batch postprocessing approach.
Citation
Pelosi, Anna; Medina, Hanoi; Van den Bergh, Joris; Vannitsem, Stéphane; Battista Chirico, Giovanni (2017-12-04). Adaptative Kalman Filtering for Postprocessing Ensemble Numerical Weather Predictions, Mon. Wea. Rev., 145, 4837-4854.
Identifiers
Type
Article
Peer-Review
Yes
Language
eng