Ensemble post‐processing using member‐by‐member approaches: theoretical aspects
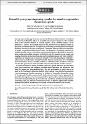
View/ Open
Authors
Van Schaeybroeck, Bert
Vannitsem, Stéphane
Discipline
Earth and related Environmental sciences
Subject
ensemble forecasting
post-processing
numerical weather forecasting
Audience
Scientific
Date
2014-05-16Publisher
Wiley Online Library
Metadata
Show full item recordDescription
Linear post‐processing approaches are proposed and fundamental mechanisms are analyzed by which the probabilistic skill of an ensemble forecast can be improved. The ensemble mean of the corrected forecast is a linear function of the ensemble mean(s) of the predictor(s). Likewise, the ensemble spread of the corrected forecast depends linearly on that of the uncorrected forecast. The regression coefficients are obtained by maximizing the likelihood function for the error distribution. Comparing different calibration approaches on simple systems that exhibit chaotic features (the Kuramoto–Sivashinsky equation, the spatially extended Lorenz system), four correction mechanisms are identified: the ensemble‐mean scaling and nudging using the predictor(s), and the ensemble‐spread scaling and nudging. Ensemble‐spread corrections turn out to yield improvement only when ‘reliability’ constraints are imposed on the corrected forecast. First of all climatological reliability is enforced and is satisfied when the total variability of the forecast is equal to the variability of the observations. Second, ensemble reliability or calibration of the ensembles is enforced such that the squared error of the ensemble mean coincides with the ensemble variance.
In terms of continuous ranked probability skill score, spread calibration provides much more gain in skill than the traditional ensemble‐mean calibration and extends for lead times far beyond the error‐doubling time. The skill performance is better than or as good as the benchmark calibration method which derives from statistical assumptions –non‐homogeneous Gaussian regression. In addition to the member‐by‐member nature of the approach, benefits compared with the benchmark method can be pinpointed. In particular, although the post‐processing methods are performed for each lead time, location and variable independently, they preserve the rank correlations and thus take dependencies across space, time, and different variables into account. In addition, higher‐order ensemble moments like kurtosis and skewness correspond to those of the uncorrected forecasts.
Citation
Van Schaeybroeck, Bert; Vannitsem, Stéphane (2014-05-16). Ensemble post‐processing using member‐by‐member approaches: theoretical aspects. , Quarterly Journal of the Royal Meteorological Society, Vol. 141, Issue 688, 807, Wiley Online Library.
Identifiers
Type
Article
Peer-Review
Yes
Language
eng