On Temporal Scale Separation in Coupled Data Assimilation with the Ensemble Kalman Filter
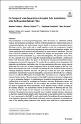
View/ Open
Discipline
Earth and related Environmental sciences
Audience
Scientific
Date
2020-03-17Publisher
Springer
Metadata
Show full item recordDescription
Data assimilation for systems possessing many scales of motions is a substantial methodological and technological challenge. Systems with these features are found in many areas of computational physics and are becoming common thanks to increased computational power allowing to resolve finer scales and to couple together several sub-components. Coupled data assimilation (CDA) distinctively appears as a main concern in numerical weather and climate prediction with major efforts put forward by meteo services worldwide. The core issue is the scale separation acting as a barrier that hampers the propagation of the information across model components (e.g. ocean and atmosphere). We provide a brief survey of CDA, and then focus on CDA using the ensemble Kalman filter (EnKF), a widely used Monte Carlo Gaussian method. Our goal is to elucidate the mechanisms behind information propagation across model components. We consider first a coupled system of equations with temporal scale difference, and deduce that: (i) cross components effects are strong from the slow to the fast scale, but, (ii) intra-component effects are much stronger in the fast scale. While observing the slow scale is desirable and benefits the fast, the latter must be observed with high frequency otherwise the error will grow up to affect the slow scale. Numerical experiments are performed using the atmosphere-ocean model, MAOOAM. Six configurations are considered, differing for the strength of the atmosphere-ocean coupling and/or the number of model modes. The performance of the EnKF depends on the model configuration, i.e. on its dynamical features. A comprehensive dynamical characterisation of the model configurations is provided by examining the Lyapunov spectrum, Kolmogorov entropy and Kaplan–Yorke attractor dimension. We also compute the covariant Lyapunov vectors and use them to explain how model instabilities act on different model’s modes according to the coupling strength. The experiments confirm the importance of observing the fast scale, but show also that, despite its slow temporal scale, frequent observations in the ocean are beneficial. The relation between the ensemble size, N, and the unstable subspace dimension, n0, has been studied. Results largely ratify what known for uncoupled system: the condition N≥n0 is necessary for the EnKF to work satisfactorily. Nevertheless the quasi-degeneracy of the Lyapunov spectrum of MAOOAM, with many near-zero exponents, is potentially the cause of the smooth gradual reduction of the analysis error observed for some model configurations, even when N>n0. Future prospects for the EnKF in the context of coupled ocean-atmosphere systems are finally discussed.
Citation
Tondeur, M., Carrassi, A., Vannitsem, S. et al. On Temporal Scale Separation in Coupled Data Assimilation with the Ensemble Kalman Filter. J Stat Phys 179, 1161–1185 (2020). https://doi.org/10.1007/s10955-020-02525-z
Identifiers
Type
Article
Peer-Review
Yes
Language
eng